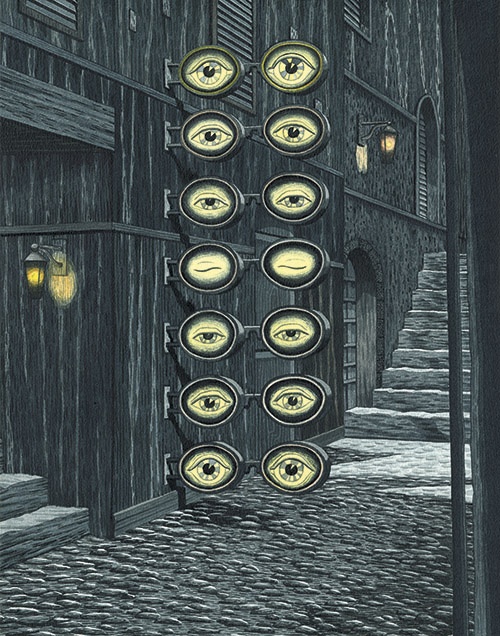
Word Search
As AI elbows its way into the translation industry, our machines—and their creators—are taking the humanity out of language.
In late 2020, Tristan Wettstein, a freelance translator based in Brittany, received a job offer to help develop a new AI tool for machine translation. At first, he was resistant to the idea. A professional translator since 2010, Wettstein had worked on technical manuals, legal contracts, academic papers and union documents, among other kinds of text. His mother was a translator too, and he was raised in both English and French, giving him an advantage in a field where professionals usually translate from one or more source languages into their mother tongue; he could go both ways, between two languages in which he felt equally at home. He enjoyed his work, the sense of artisanship he felt rewriting one language into another, careful to preserve the meaning and spirit of the original—the way an art restorer may think of their profession as something of an art itself.
But, during his decade in the translation industry, Wettstein had noticed a disheartening trend. As machine translation grew more sophisticated, the clients who once employed him for original work were increasingly sending him texts that had already been translated, for free, by AI tools. His job in these cases was to fix the AI’s mistakes—and there were always mistakes. These were typically small (such as translating the IT term query to French as demande rather than the more appropriate requête), making them difficult to catch but simple enough to correct. Some problems, however, were more fundamental. “Language is such an expression of human variety, of human dynamism,” Wettstein says. “And that’s not something that an AI can learn until it understands—not just ‘can parse out in a logical way’ but understands—the context and the intent and the meaning of a text beyond its words.” Even after he had made it comprehensible, the writing remained stiff and unnatural, verbose without voice. Sure, his clients weren’t after poetry, but the content he sent back was still intended to be read.
“There’s an alienation,” Wettstein says. “You don’t feel like there’s as much of yourself in it, and you’re not putting forward your best work because it’s not entirely of your own agency.” Other translators avoid working with the output of their machine counterparts, a task known as post-editing, entirely. For Sophie Boivin-Joannette, an English-to-French translator based in Montreal, “it feels like parts of the industry are heading toward humans being tasked with less creativity and critical thinking. More and more, we’re just checking the work of the AI.” Boivin-Joannette feels fortunate to have avoided post-editing in her career, primarily because “it pays like shit”—rates are typically around half of what they would be for original translation.
“You’re doing comparative editing without necessarily having access to the source text, so it’s a lot of guesswork,” says Amie Monroe, a co-founder of the Montreal-based translators’ cooperative Coop l’Argot, which offers translation between English, French and Spanish. “Like, what did this robot believe it was saying? Or what was this robot trying to express?” Monroe refuses to post-edit on ethical grounds: the massive bilingual datasets used to develop a machine translator represent the work of an untold number of uncredited human translators, taken and often profited from without their consent. “I also have an issue with making use of a technology that has some serious flaws in terms of quality and that is sort of designed to put us out of work in the long term.”
It may seem surprising, then, that in the end, Wettstein took the job. He spent months translating snippets of informal, SMS-style text (“bb get some milk on ur way home kthx”) from English to French in order to help train ISAAC, an AI language-learning tool developed by Germany’s University of Tübingen, with funding from German government agencies. When I mention that training ISAAC seems to make him somewhat complicit in his field’s predicament—declining wages as post-editing becomes increasingly popular—Wettstein points out that his refusing the gig would hardly have changed the course of industry trends. He had worked on the project alongside thousands of other freelance translators, none of whom he ever met. Plus, it had been a dry month, and a low-paying job was better than nothing. He was also curious about what went into the creation of a machine translator. “When you’re not directly involved in it,” he says, “it can be hard to measure how these AIs are getting trained and why their outputs are the way they are.” Training ISAAC had its upsides—Wettstein gained a better understanding of AI translators and is now more productive when working with them—and it was refreshing that, for once, he had been given a choice.
The growing language skills of computers have been a hot topic lately, which has come with the realization that, like it or not, we’ve all been enlisted in the AI revolution. The content of our digital lives, from social media to works of art, has been scraped into databases, broken down and analyzed to help computers convincingly mimic a variety of tasks that were once exclusively human: writing an email, painting a portrait, composing a song. Users of AI image generators have reported finding what appear to be watermarks and artists’ signatures scattered throughout their results like copyrighted hairs in their soup. And the legal pushback from the content side has begun: in February, for instance, US-based media company Getty Images sued Stability AI, the developer behind one of these tools, for $1.8 trillion USD. The following month, the UK committed to developing a code of practice for AI firms that would delineate the kinds of data they can and cannot use, attempting to balance technological innovation with the rights of creative workers.
When it comes to translation, this kind of aggregate plagiarism is often considered fair use: in an increasingly globalized information economy, the public benefits of widespread access to AI translators are not hard to imagine. The problem arises as these tools creep from the pockets of well-meaning tourists and spread, by virtue of their convenience, to where they don’t belong. The work of commercial translation is increasingly becoming less a text produced than a service rendered, but translation is also, inescapably, writing: interpreting and expressing fixed concepts in slippery symbols. For many translators, the spread of AI—and the labour dynamics of their forced partnerships with it—has cheapened their craft, drained their jobs of satisfaction and led to a proliferation of bad writing.
“We’re really preoccupied as translators with the idea of wanting to communicate between people, not just productivism, the ideology of producing or just making money,” Wettstein says. “I think it’s important to understand that the industry isn’t necessarily just how it’s used as a tool. It also has a life of its own.”
The automation of labour—or, rather, the fantasy of automating labour—is hardly new, nor is its accompanying hype. In “Calibrating Agency: Human-Autonomy Teaming and the Future of Work amid Highly Automated Systems,” their 2019 paper on AI and automation, Melissa Cefkin, Lee Cesafsky and Erik Stayton of Silicon Valley’s Alliance Innovation Lab trace this rhetoric back to the 1780s, when American inventor Oliver Evans was promoting his “fully automatic” flour mill—and downplaying the roles of the humans who oversaw, maintained and directed the machine’s operations, without whom it wouldn’t work. “While the business rhetoric around AI, machine learning, and predictive analytics argues that human beings can be eliminated from a wider and wider range of tasks,” the authors write, “we know from a long history of automation studies that the reality is never this simple. Human roles and agencies are displaced, shifted in time and space, but not simply eliminated or made obsolete.”
Still, the history of machine translation, which is about as old as computers themselves, has been accompanied by the dream (or dread) of replacement. In 1954, in what the New York Times heralded as “the culmination of centuries of search by scholars for a mechanical translator,” IBM and Georgetown University held a public demonstration of an automated Russian-to-English converter: an operator punched in Russian phrases, and a few seconds later, the machine spat out their English equivalents, such as “Starch is produced by mechanical method from potatoes” or “We transmit thoughts by means of speech.”
In a now-familiar cycle, the media and public seized upon this new technology to fuel breathless speculation. “Five, perhaps three, years hence,” one professor mused to the Christian Science Monitor shortly after the demonstration, “interlingual meaning conversion by electronic process in important functional areas of several languages may well be an accomplished fact.” Neil Macdonald, an editor at the journal Computers and Automation, went further in describing the technology’s implications: “Linguists will be able to study a language in the way that a physicist studies material in physics, with very few human prejudices and preconceptions.”
Less foregrounded in the reporting on the Georgetown-IBM demonstration was the program’s 250-word vocabulary, or the fact that the sentences it translated had been designed for the task—avoiding, for example, terms that can have different meanings depending on the context in which they’re used. It was, after all, the height of the Cold War, and the language of the Soviets was treated as an enemy code to be cracked, not the independent, living distillation of centuries of culture.
For Lynne Bowker, a professor of translation at the University of Ottawa who has studied machine translation for decades, the discourse surrounding the tech is characterized by a lack of nuance. After all, “machine translation” is itself a generalization, a blanket term for dozens of programs attempting to translate between tens of thousands of unique language pairs with varying degrees of success. Overall, the responsible use of these tools begins by recognizing their limitations. “Machine-translation literacy has become increasingly important because the technology has left the realm of language professionals and is now in the hands of everyone,” Bowker says. “It’s so easy to use that it gives the wrong impression. It gives the impression that translation is easy.”
But translation is difficult, especially for machines. “Computers are really good at math, really good at pattern matching, but not really good at understanding how the world works,” Bowker says. “Computers don’t understand anything, really. They don’t have knowledge, per se.” People may set out to learn a new language by studying its vocabulary and grammar, but to achieve fluency requires transcending these rules and immersing oneself in its social and cultural circumstances. “The computer is at a real disadvantage when it’s trying to process language the way that people do, because we don’t just use grammar and dictionaries—we actually use our knowledge of the world.”
Georgetown and IBM’s rule-based approach, with its rigid parallel vocabularies and linguistic diagrams, turned out to be a dead end. In a 1966 report, the US Automatic Language Processing Advisory Committee called machine translation expensive, inaccurate and unpromising. Language wasn’t math, couldn’t be treated as programming and always proved too variable, too messy, alive. It could, however, be reduced to data.
In the early 1990s, the field of machine translation was revived by a paradigm shift: computers didn’t have to understand natural languages, they just had to seem to. With enough parallel bilingual text to analyze, they could parrot back translations relatively accurately by calculating which sets of words in one language are most likely to correspond with which sets of words in another—essentially, a system of educated guesswork. As Christine Mitchell wrote in an article for the Walrus last year, IBM’s first breakthrough in this big-data model of machine translation was unwittingly helped along by the Canadian government: in the mid-1980s, a computer-readable magnetic tape reel arrived at the company’s New York headquarters from an unknown sender. It was a part of the parliamentary proceedings record, Hansard, containing fourteen years of transcripts—millions of words of parallel text in English and French. Several years later, IBM unveiled a new statistical model for translation from French to English, citing Hansard as an essential part of its development, much to Canada’s surprise.
Now, as the world’s data volume is tallied up in zettabytes, or trillions of gigabytes, we’re inundated with ever-more-sophisticated neural AI language models, and the Hansard story seems prophetic—an early lesson to mind the origins of the data these tools are fed. Compared with the wholesale web scrapings that programs like ChatGPT use, the pre-translated parallel texts needed to train machine translators are a scarcer resource, so international bodies such as the European Union maintain publicly available collections of them in order to aid research and facilitate communication between member states. But framing bilingual data as a public utility overlooks the professionals from whose labour it springs. “The data is not just drifting around out there to be harvested. A skilled translator has created it,” Bowker says. “Their own data has been taken from them without their permission, and now it’s being used to push down their prices.”
Economic principles suggest that, generally, as demand for a service increases, the workers who provide it should earn more. And globalization has created high demand for commercial translation, with the US Bureau of Labor Statistics expecting the employment of interpreters and translators to expand by 20 percent from 2021 to 2031. In 2001, English linguist William John Hutchins saw machine translation as a force to help propel this growth, writing in the International Journal of Translation that people who use the “crude output” of machine translation systems will come to realize the “added value (that is to say, the higher quality) of professionally produced translations.” He believed that this would lead to a rise in demand for human translation, that “automation and MT will not be a threat to the livelihood of the translator, but will be the source of even greater business and will be the means of achieving considerably improved working conditions.”
The gig economy has since destroyed this expectation. When those demanding your services are large corporations, they tend to find a way to pay less, such as by using the disorganized and disparate labour of underpaid contract workers—or by using technology to push humans to the margins. As translation increasingly becomes a freelance profession, intermediary agencies continue to insert themselves between clients and gig workers earning lower and lower rates. In a recent interview with the Hollywood Reporter on the US writers’ strike, corporate consultant Amy Webb described executives in the TV and film industry asking how quickly they could bring in an AI tool to generate their scripts. “And they’re serious,” Webb emphasized. “The conditions are right in certain cases for an AI potentially to get the script 80 percent of the way there and then have writers who would cross the picket line do that last 20 percent of polishing and shaping.” This process of post-editing is designed to undermine the market value of human creativity.
Matt Hauser, a senior vice-president at TransPerfect, one of the world’s largest translation agencies, argues that the spread of machine translation has resulted in more opportunities for translators—but those opportunities are largely in post-editing. Hauser, describing a process his company calls “human-in-the-loop” translation, echoes Webb’s grim forecast for the future of screenwriting. “We can use machine translation to get it close,” he says, “and then we can use humans to get it exactly where it needs to be. Because the needs of every client are a little different, you know—brand voice and things like that.”
Human-AI labour partnerships come in various configurations, some more conducive to worker satisfaction and quality of output than others. The 2019 paper on AI and automation, “Calibrating Agency,” opens with the example of a remote operator monitoring the progress of a self-driving car. The vehicle encounters an obstacle that it cannot circumvent without crossing the road’s double yellow line, so it awaits the operator’s input based on her contextual knowledge of the situation, which might include the jurisdiction’s traffic laws or the body language of pedestrians. This kind of relationship between operator and AI, called “human-autonomy teaming,” is a delicate balance of agencies, the human’s and the machine’s.
But it’s very different from the working relationship between the post-editor and AI. Rather than collaborating with the machine, the editor hurries after it, repairing the signposts it has run down.
“Today, there are some areas where clients are not comfortable with [machine translation],” Hauser admits. When it comes to texts like pharmaceutical labels or legal documents, small errors can have dire consequences. In Rest of World, an online global tech publication, crisis translator Uma Mirkhail tells the story of a Pashto-speaking refugee from Afghanistan whose asylum claim was rejected by a US court because of discrepancies between her written application and the account she’d given in interviews: she had described being alone during a particular event, but an AI tool had mistranslated her first-person singular pronouns as “we.”
Context-dependent references such as pronouns are a constant struggle for machine translators, and in many cases, these errors follow an unfortunately familiar pattern. Until late 2018, Google Translate would regularly convert gender-neutral pronouns from one language into gendered pronouns in another. Gender biases also presented in more specific ways—words like doctor and strong became disproportionately associated with he/him pronouns while nurse and beautiful were often correlated with she/her. As US linguist Andrew Garrett pointed out in April, ChatGPT displays a similar ignorance: when prompted to identify “the professor” and “the graduate student” in a series of sentences containing one ambiguous “she” pronoun (“The professor married the graduate student because she was pregnant”), an AI tool consistently failed to recognize the possibility that the professor could be a woman.
The demographics of the industry behind these tools seem relevant here: 64 percent of high-tech employees in the US are men and 68.5 percent are white, according to a 2014 report from the US Equal Employment Opportunity Commission—which might help explain how these biases were overlooked until users from the wider public began calling attention to them. But the data on which AI tools are built extends far beyond those doing the actual building. When we attempt to train an algorithm by drawing from the raw sum of digitized text in recorded history, we may succeed in teaching it to imitate our languages, but we also encode it with our faults. Prejudices we’re struggling to overcome instead become entrenched. An oft-cited example of this in action comes from the New Jersey criminal justice system, which began using AI in 2017 to provide “objective” risk assessment and help set bail conditions. Instead, the technology was found to have to have perpetuated long-standing racial disparities in who is jailed before they get a trial.
This doesn’t mean that AI is or can be racist or sexist—even that would credit it with too much consciousness—it just means that racism and sexism exist, and the wide-net approach of big data tends to pick them up. AI may be forgiven, for unlike us, it knows not what it says. But the tech’s boosters (who wonder, “Is the world ready for ChatGPT therapists?”) seem keen to ignore this fact. Meanwhile, reminders abound. For example, there are what the industry, in its slyly humanizing way, has dubbed “hallucinations”: random strings of output text produced by AI models, some gibberish, some lifted directly from training corpuses. In Wettstein’s experience, these outbursts are rare in machine translation, more likely to occur as programs struggle with figurative or embellished passages—the kind of literary writing that commercial translators don’t often encounter—but they do happen. In a 2021 study of the phenomenon, an AI tool tasked with a German sentence for which a correct English translation would have been “This can only be detected if controls undertaken are more rigorous” instead seemed to draw on the words of Italian dictator Benito Mussolini: “Blood alone moves the wheel of history, I say to you and you will understand, it is a privilege to fight.”
Still, as Wettstein points out, there is currently more demand for translation services than there are human translators to handle it, so machine translation “is bound to find its niche.” Meanwhile, Hauser, whose company has developed its own translation AI, seems downright enthusiastic about the technology’s spread (or, as he puts it, “applying technology in every facet of our business in the interest of making sure we’re driving efficiencies and maximizing productivity while lowering costs for customers”) and believes that no sector is immune. “As these technologies start becoming more and more ubiquitous and you’re building bigger datasets, I think eventually the reticence on the part of high-risk industries will slowly go away,” he says, making no mention of whether it should.
Isadore Toulouse is a member of the Wiikwemkoong Unceded First Nation on Manitoulin Island in Ontario and grew up speaking Anishinaabemowin. Starting in grade one, English was imposed upon Toulouse. “I was told at a young age that I would not get anywhere in life if I continued to use my language,” Toulouse says, “by the nuns and missionaries who were part of our community back then.”
The nuns and missionaries, it turned out, were wrong. For the past thirty-two years, Toulouse has made a living translating between English and the Anishinaabemowin language as well as teaching the methodology of Anishinaabemowin education at Lakehead University, training generations of teachers and working to preserve Anishinaabe culture after centuries of suppression. From the 1880s to the late 1990s, the governments of Canada and the US, as well as the Roman Catholic, Anglican, Methodist, Presbyterian and United churches, forced Indigenous children into residential and boarding schools, where, among other abuse, they were punished for speaking their mother tongues. Today, Michigan State University estimates that there are roughly 36,500 Anishinaabemowin speakers remaining in Canada and the US, and far fewer have the fluency to pass it on. “We need to preserve, maintain and revitalize our language,” Toulouse says, “or this is where it ends.”
Like many Indigenous languages, Anishinaabemowin is an oral tradition. While symbols were used to communicate sacred teachings, the most widespread written form emerged when missionaries began transcribing them phonetically using the Latin alphabet—less for language preservation than to aid in their mission of Christian conversion. But “language and culture cannot be separated,” says Toulouse. “You cannot teach language without culture; you cannot teach culture without language.” Now, the romanized writing system is being used to safeguard Indigenous languages in an era when text has usurped speech. With each publication, Indigenous-language writers and translators are building out their own database—and, in the age of AI, it’s one they will need to protect.
“I’ve heard it said that data colonization is the final colonization,” Te Taka Keegan, a computer scientist working to preserve the Māori language, said at a 2019 conference on Indigenous language revitalization, as reported by the Tyee. He went on to describe a six-month stint helping Google develop a Māori translation tool. Years after completing his work on the project, he noticed that the English translations of Māori phrases were changing: “Tenei au ka mihi atu ki a koutou katoa,” for instance, went from “Today I greet you all” to “I would like to thank you all.” Most of the time, Keegan said, these shifts were “not for the better.” And, more troublingly, they seemed to involve no human input—much less input from Māori people. “The way the system is set up, it automatically gathers data; it automatically makes the change.”
“To be honest,” Keegan added, “no one [at] Google really cared about the Māori language. The people that care about the Māori language are the people that speak the Māori language. If we want to create technologies for our own language, we have to do it ourselves.”
Last September, OpenAI, the AI research company responsible for ChatGPT, launched Whisper, an audio translation and transcription program that includes the Māori language. In the following months, community members raised concerns about where exactly its data—1,381 hours of speech scraped from the web—had come from. Datasets for Indigenous languages are still relatively small, making them susceptible to pollution by irrelevant or low-quality content, known as noisy data. “Data is like our land and natural resources,” Māori ethicist Karaitiana Taiuru told the Thomson Reuters Foundation following Whisper’s release. “If Indigenous peoples don’t have sovereignty of their own data, they will simply be re-colonized in this information society.”
In the 2022 paper “Translation as Discrimination,” York University linguist Philipp Angermeyer writes of machine translation as working hand-in-hand with what he calls “punitive multilingualism,” a system that, by tying translation quality to the wealth of available data, punishes those living outside of a society’s dominant language (and reinforces that dominance). For example, Angermeyer points to his research on the public order signage of Parkdale, a Toronto neighbourhood which, around the late 2000s and early 2010s, had a significant population of Hungarian-speaking Roma—members of a traditionally nomadic culture with a long history of persecution, including mass murder in the Holocaust and recent efforts by the Canadian government to prevent their immigration. For a 2017 paper, Angermeyer documented the few dozen signs in Parkdale (such as a public building’s code of conduct) for which translation into Hungarian had been posted. Beyond the dim view implied by the number of prohibitory signs, he found that many contained ungrammatical Hungarian produced using Google Translate. His Roma interviewees described the signs as varying from incomprehensible to impolite. Some felt the language stereotyped them as likely troublemakers, others as if, by communicating with them via the garbled output of a free online tool, the city was trying to avoid interacting with them or their culture in a meaningful way.
To Bowker, the poor quality of minority-language machine translation makes the technology’s spread all the more concerning. It also risks flattening the regional dialects of global languages. Translating into English, for instance, operators can occasionally choose between US and UK variants, but what about Irish English, Jamaican English, or African-American English, each of which represents a cultural tradition with its own literature? To AI, they’re too small to matter, outliers in the melting pot of our collective data. “These tools will further marginalize the languages that are already at the margins,” Bowker says—along with the people who embody those languages.
The utilitarian logic of big tech and global commerce would seem to prefer that we all speak the same handful of data-rich languages, but the human impulse is the opposite: communities are constantly customizing and adapting their languages—even within the same languages spoken around the world, even the languages imposed by colonizers centuries ago—to define and distinguish themselves. “People are proud of their own language,” Bowker says. “It’s connected to your culture. It’s connected to your family. It’s connected to life in ways that go beyond a purely functional, transactional tool.” If we allow the automation of language work to continue on its present course, we risk more than the craft of translation or the art of writing: we risk the humanity of language itself. When we remove language from human hands, not only do the machines we entrust it to fail to understand it; we fail to understand it, too. ⁂
Jonah Brunet is a writer and editor based in Montreal. His work has appeared in the Walrus, This and Toronto Life, where he is currently the copy editor.